Video: an introduction to Work Package 3 ‘Mining Musical Patterns’
Relying on the results of WP2 -ontology-based knowledge graphs- the overall goal of WP3 is to devise approaches to analysing large repositories of music (tunes, songs, etc.) to identify common, meaningful patterns that are indicative of their identity, filiation or cultural association (genres, origin, etc). Work package leader James McDermott explains the ‘Mining Musical Patterns’ work of WP3 in our latest video on YouTube.
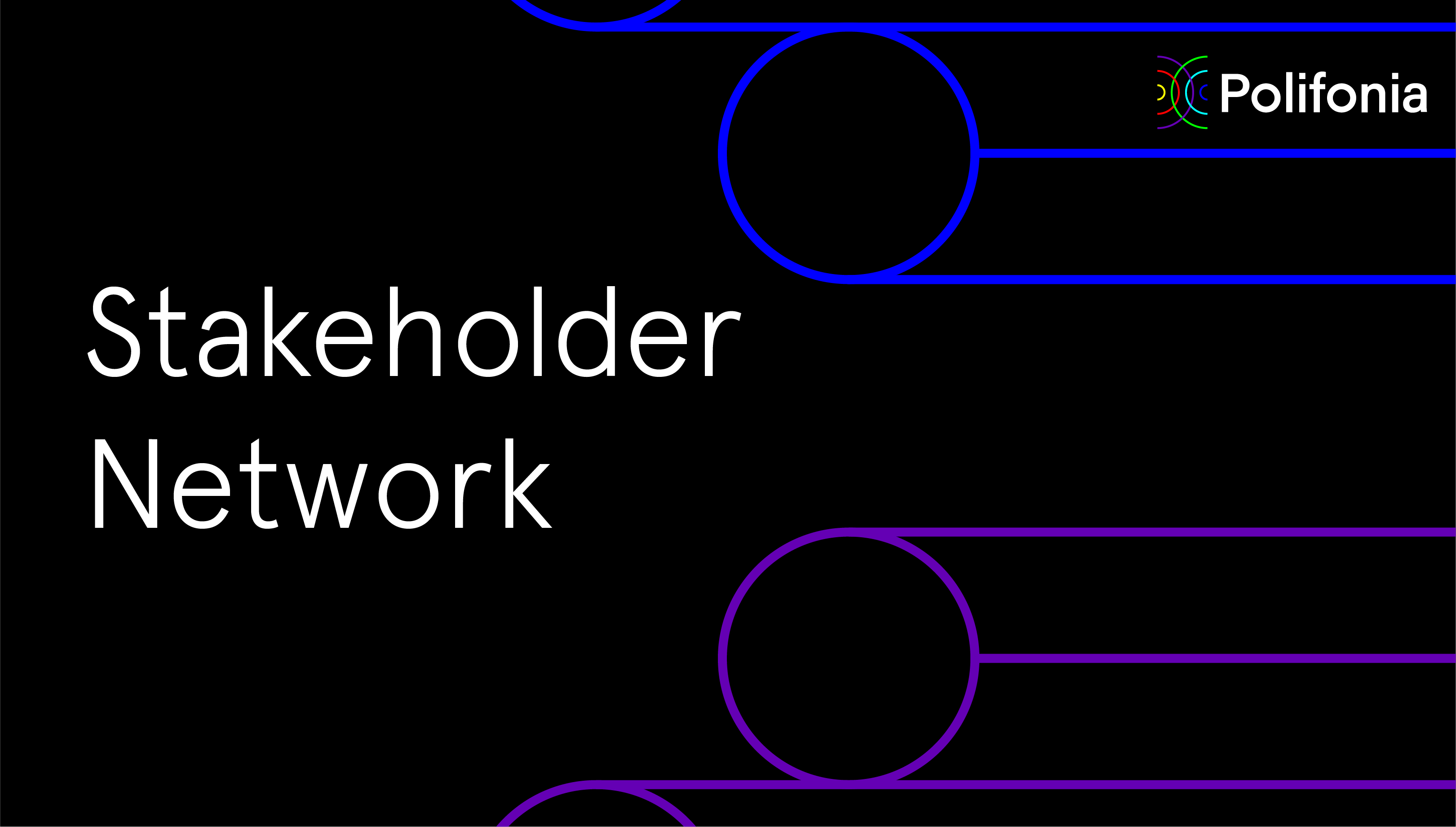
Relying on the results of WP2 -ontology-based knowledge graphs- the overall goal of WP3 is to devise approaches to analysing large repositories of music (tunes, songs, etc.) to identify common, meaningful patterns that are indicative of their identity, filiation or cultural association (genres, origin, etc). Work package leader James McDermott explains the ‘Mining Musical Patterns’ work of WP3 in our latest video on YouTube.
About Music Information Retrieval
Pattern extraction is part of the interdisciplinary science of Music information retrieval (MIR). MIR was born from computational musicology in the 1960s. MIR combines machine learning with expert human knowledge to use digital music data – images of music scores, “symbolic” data such as MIDI files, audio, and metadata about musical items – for information retrieval, classification and estimation, pattern finding or sequence labeling. Major industry players such as Soundcloud, Spotify and Deezer heavily rely on MIR technology, to support their genre-classification or recommender systems. Musicologists use MIR, for instance pattern recognition, to test theories on ‘cultural cross-pollination’.