Can a computer generate realistic music?
Many people would probably answer this question with a clear “no”. Is it because they really believe computers cannot? Or maybe there is an inner voice that refuses to believe that musical creativity is not exclusive to humans? In the meantime, AI systems such as DALL-E and ChatGPT have already proven unprecedented performance for generating images and textual artefacts, and are now becoming ubiquitous in our society. What about AI-generated music?
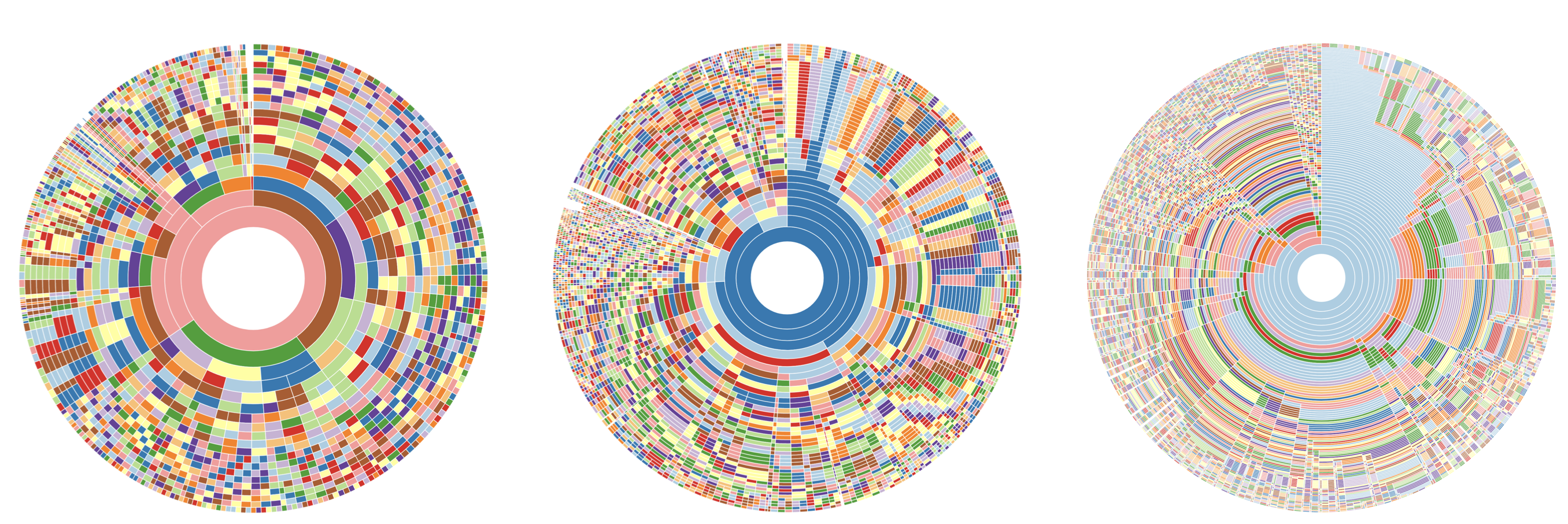
by Jacopo de Berardinis & Max Tiel
Many people would probably answer this question with a clear “no”. Is it because they really believe computers cannot? Or maybe there is an inner voice that refuses to believe that musical creativity is not exclusive to humans? In the meantime, AI systems such as DALL-E and ChatGPT have already proven unprecedented performance for generating images and textual artefacts, and are now becoming ubiquitous in our society. What about AI-generated music?
The automatic composition of music dates back to the ancient Greeks, and remained up to and beyond Mozart with the “Dice Game”. Even Ada Lovelace, an esteemed mathematician, speculated that the “calculating engine” might compose elaborate and scientific pieces of music of any degree of complexity or extent. In recent years, AI systems have achieved remarkable results on symbolic and audio music [1]. The variety of computationally creative methods for music is quite broad and diversified, and has already enabled the exploration of novel forms of artistic co-creation [2]. These range from the automatic generation, completion, and alteration of chord progressions and melodies, to the creation of mashups, and audio snippets from textual prompts [3].
However, music AI systems are still far from generating full musical pieces that can be deemed realistic across several musical dimensions (harmony, form, instrumentation, etc.). First, most systems require hours and hours of human-composed (real) music before they can start generating interesting artefacts. Most importantly, although the machine can explore thousands of musical ideas, the resulting compositions are rarely used as final outputs. To make these “musical drafts” realistic, human intervention is often needed to correct, adapt, and extend the generations – depending on the creative workflow put forth by the artist. Hence, human involvement is at least required upstream (data curation) and downstream (music adaptation) of the generation process.
Human participation is also needed to evaluate music generation systems in a variety of forms, ranging from Turing tests to musicological evaluations. Recently, researchers are also starting to devise new computational methods to automate this process. This way, every time a new music generation system is developed, its outputs can be coherently measured and compared in a controlled framework. Towards this direction, Dr. Jacopo de Berardinis, postdoc at King’s College London, has recently published a new method on evaluating the structural complexity of machine-generated music: by letting an algorithm decide on whether a piece of sound has realistic musical structure or not. De Berardinis is part of the Polifonia consortium, an AI-music project funded by the European Union’s Horizon 2020 research and innovation programme.
De Berardinis: ‘’composing musical ideas longer than phrases is still an open challenge in computer-generated music, a problem that is commonly referred to as the lack of long-term structure in the generations. In addition, the evaluation of the structural complexity of artificial compositions is still done manually – requiring expert knowledge, time and involving subjectivity which is inherent in the perception of musical structure.’’
AI can create short pieces of music or make variations and interpolations on existing pieces. But at some point the music will start diverting because machine learning models still struggle with long term dependencies. By automating the process of the evaluation of musical output, a lot of resources that are in human analysis of the music data, can be saved. To address this, de Berardinis detects musical structures from the sound [4], and describes their decomposition process [5]. This then allows the system to ‘judge’ the structural complexity of music on a scale from ‘real music’ to ‘random music’. In other words: you provide a music dataset and let the system match the input music on a continuum between these two complexity classes.
In conclusion, the automatic generation of music that cannot be distinguished from human compositions still remains an open challenge for AI music research. In the meantime, opening a debate on the eventual implications of these methods is also necessary – as having a system that can realistically generate music raises ethical concerns. Instead of designing tools that could potentially replace artists and composers (for certain commissions), de Berardinis argues that research should focus on leveraging the generative capabilities of AI models to design new systems that can enhance and augment the creative potential of artists – thereby enabling novel opportunities for Artificial Intelligence Augmentation (AIA) [6]. With these concerns and objectives, a team in Polifonia is currently working towards the creation of resources and algorithms to promote more transparent, fair, and reliable paradigms in music AI.
[1] Briot, J. P., Hadjeres, G., & Pachet, F. D. (2020). Deep learning techniques for music generation (Vol. 1). Heidelberg: Springer.
[2] Huang, C. Z. A., Koops, H. V., Newton-Rex, E., Dinculescu, M., & Cai, C. J. (2020). AI song contest: Human-AI co-creation in songwriting. arXiv preprint arXiv:2010.05388.
[3] Agostinelli, A., Denk, T. I., Borsos, Z., Engel, J., Verzetti, M., Caillon, A., … & Frank, C. (2023). Musiclm: Generating music from text. arXiv preprint arXiv:2301.11325.
[4] de Berardinis, J., Vamvakaris, M., Cangelosi, A., & Coutinho, E. (2020). Unveiling the hierarchical structure of music by multi-resolution community detection. Transactions of the International Society for Music Information Retrieval, 3(1), 82-97.
[5] de Berardinis, J., Cangelosi, A., & Coutinho, E. (2022). Measuring the structural complexity of music: from structural segmentations to the automatic evaluation of models for music generation. IEEE/ACM Transactions on Audio, Speech, and Language Processing, 30, 1963-1976.[6] Carter, S., & Nielsen, M. (2017). Using artificial intelligence to augment human intelligence. Distill, 2(12), e9.